- Worked with the largest regional bank in the South-East USA which spends a considerable amount of time and resources investigating 30k+ suspicious money laundering alerts per month to develop a model which predicts the seriousness of the alerts.
- Led a team of 4 and tackled issues such as peer – profiling, unavailable data and false positives by feature generation and selection.
- Tested the data with Logistic Regression, Random Forest, SVC and ADA Boost models to predict the quality of alert (High or Low) using precision and recall parameters.
- Delivered the ADA Boost model with a precision of 38.50% and recall of 78.48% for its high recall prediction.
Photo on InternationalBanker.com
Anti Money Laundering
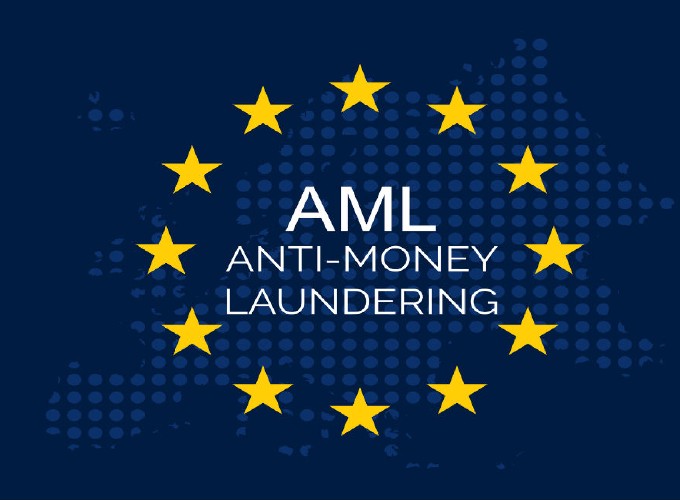